An Executive Guide to Understanding the 2024 Nobel Prize in Physics.
- Fernando Negrini
- Oct 9, 2024
- 6 min read
Updated: Apr 7
The 2024 Nobel Prize in Physics was awarded to John Hopfield and Geoffrey Hinton for their pioneering work in modern machine learning using artificial neural networks. Their contributions have transformed AI technologies and highlight the intersection of physics and AI, showing how fundamental scientific principles can drive technological advances.
This award also emphasizes the impact of interdisciplinary research.
By integrating ideas from physics, biology, and computer science, Hopfield and Hinton demonstrated how diverse fields can solve complex problems. Their work paved the way for adaptive systems that solve issues in healthcare, finance, and industry, underscoring the importance of cross-disciplinary collaboration.
Their success illustrates how understanding fundamental concepts in one field can lead to breakthroughs in another. By using physics principles to create neural network models, they showed how theoretical knowledge can become practical applications with significant societal impact. This blending of disciplines expands how we use scientific research and often leads to innovative insights.
Who Are the Laureates?
John J. Hopfield: Born in 1933, Hopfield earned his PhD in Physics from Cornell University in 1958 and is currently a Professor at Princeton University. He is known for his work connecting physics with neural computation, making significant contributions to artificial neural networks and our understanding of brain processes.
Geoffrey E. Hinton: Born in 1947, Hinton completed his PhD in Artificial Intelligence in 1975 (as presented in his CV) from The University of Edinburgh and is a Professor at the University of Toronto. Known as one of the "godfathers of deep learning," Hinton's work on backpropagation and deep neural networks has been foundational for AI technologies like virtual assistants and autonomous vehicles. He has also been an advocate for the ethical use of AI.
Key Contributions Leading to the Nobel
John Hopfield developed the associative memory model, known as the Hopfield network, in the 1980s. This model uses energy minimization concepts from physics to store and reconstruct data patterns, laying the groundwork for efficient information retrieval systems.
Geoffrey Hinton expanded on Hopfield's work with the Boltzmann machine, using statistical physics to teach neural networks to identify data properties autonomously. His work on deep learning and backpropagation helped overcome challenges in earlier AI systems, enabling scalable and practical applications. These contributions launched the modern era of deep learning, which underpins many AI technologies today.
The Impact of Their Work Today
Hopfield and Hinton's work is foundational to many AI applications, including image and speech recognition, autonomous driving, and advanced material research. Their models are used to solve problems across disciplines, from medicine and finance to creative industries.
In physics, neural networks are used to discover new materials, optimize quantum systems, and advance predictive capabilities.
Their work has influenced reinforcement learning, generative AI, and unsupervised learning, making neural networks indispensable tools in addressing real-world challenges.
The Controversy and Debate
The awarding of the Nobel Prize to Hopfield and Hinton has sparked significant controversy. Critics argue that the focus on artificial intelligence represents a shift towards celebrating popular technologies rather than fundamental physics discoveries. They question whether the work of Hopfield and Hinton falls more appropriately under computer science or engineering rather than physics.
The critics claim that while their work uses principles from physics, it does not represent a direct advancement in our understanding of the physical universe in the traditional sense of physics, such as quantum mechanics or astrophysics. This perspective raises concerns about the direction of the Nobel Committee, suggesting it might prioritize current trends and popular appeal over groundbreaking but less widely understood physics discoveries.
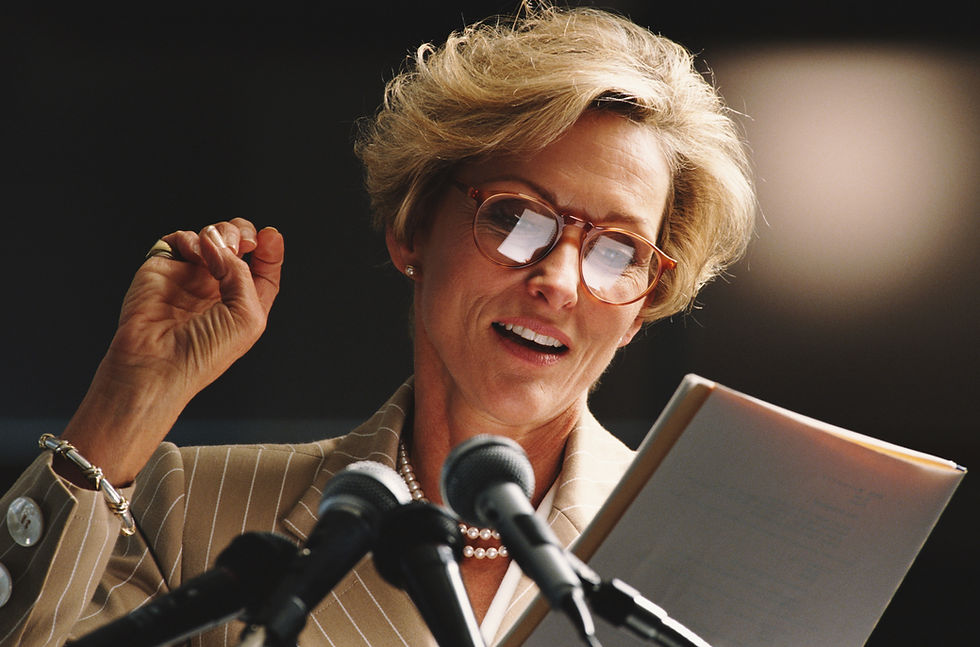
Another concern is whether awarding the prize to AI research may undermine recognition of other fundamental breakthroughs in physics, such as advancements in quantum theory or cosmology, which remain underappreciated compared to the more publicly visible advances in AI. Critics fear this trend could lead to the neglect of core areas of physics research that are crucial for our broader understanding of the universe.
Supporters, on the other hand, argue that the contributions of Hopfield and Hinton are deeply rooted in physics. Their models apply physical principles such as energy minimization and statistical mechanics to describe how neural networks learn and evolve.
The blending of physics and computation in their work represents a natural extension of the discipline into new areas, broadening the impact of physics on other domains and demonstrating the flexibility and applicability of physical concepts.
In this sense, their work has advanced our understanding of emergent behaviors in complex systems, which is a core goal of physics.
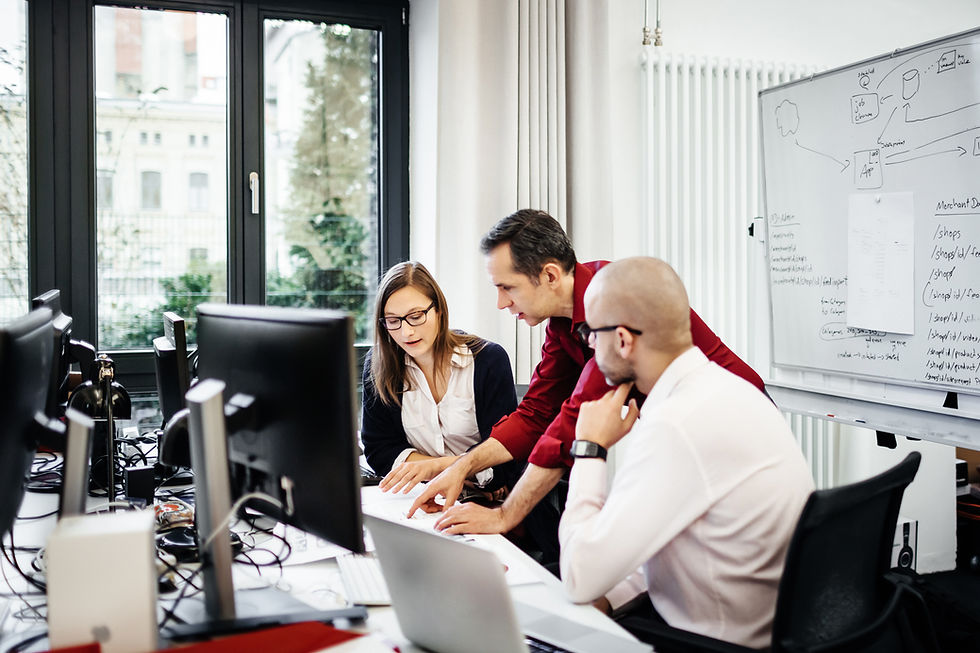
Furthermore, the application of AI in physics is transforming both theoretical and experimental research. AI techniques are now crucial for making predictions, uncovering data patterns, and simulating complex systems that were previously impossible to model accurately or efficiently. Supporters emphasize that Hopfield and Hinton’s work has fundamentally changed the practice of physics, enabling physicists to approach problems in entirely new ways.
AI also aids in experimental physics, such as the search for the Higgs Boson, where machine learning techniques are used to classify events and distinguish between signal and background noise, significantly enhancing the efficiency of data analysis at large experiments like those conducted at CERN.
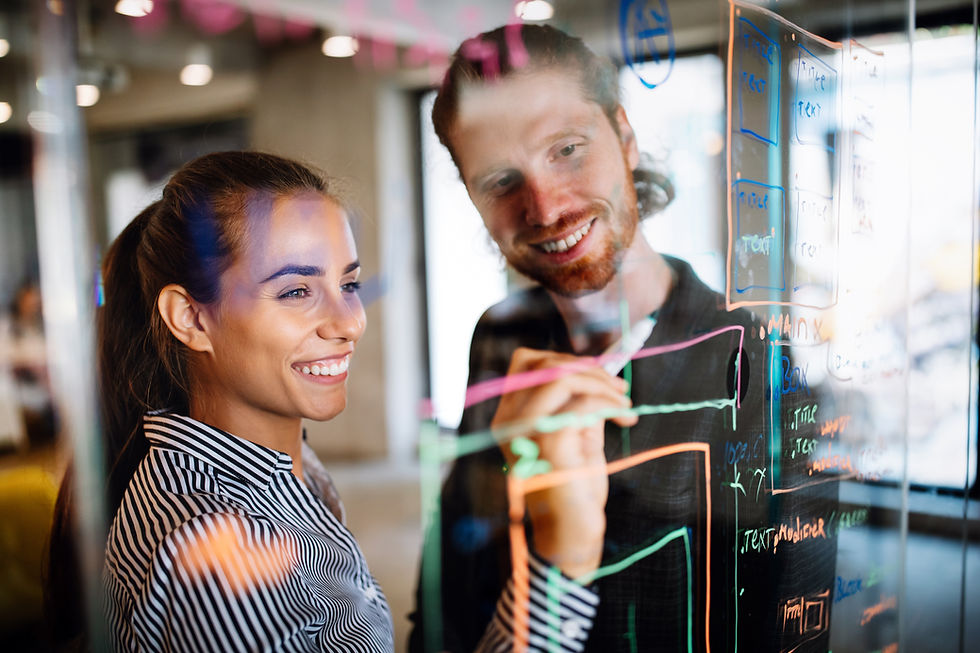
By enabling these new methods, Hopfield and Hinton’s contributions are viewed as a powerful demonstration of how physics can evolve through the integration of computational tools, which are increasingly necessary for exploring the complexities of the universe.
Supporters further argue that interdisciplinary approaches are the future of scientific progress. By recognizing contributions that bridge multiple fields, the Nobel Committee is encouraging researchers to explore the intersections of different disciplines, where some of the most impactful innovations are likely to emerge.
The use of AI in physics exemplifies how integrating computational techniques with traditional scientific inquiry can lead to breakthroughs that benefit not only the scientific community but also society at large.
Applications of AI in Physics
AI has proven to be a powerful tool in advancing various areas of physics. Here are a few notable examples:
Black Hole Simulations: AI is being used to simulate black hole accretion flows, providing new insights into the behavior of matter under extreme gravitational forces. Traditional numerical methods often struggle with the complexity and scale of such simulations, but AI models can offer faster and more accurate predictions. (Nemmen, R., Duarte, R., & Navarro, J. P. (2020). The first AI simulation of a black hole.)
Symbolic Regression for Physics Discovery: A computational framework for physics-informed symbolic regression has been used to integrate domain knowledge and discover meaningful symbolic expressions in experimental data. This helps physicists derive new relationships and better understand complex systems. (Keren, L. S., Liberzon, A., & Lazebnik, T. (2023). A computational framework for physics-informed symbolic regression with straightforward integration of domain knowledge).
Higgs Boson Discovery: Machine learning methods, including logistic regression, decision trees, and gradient boosted trees, have been applied to solve classification problems related to the Higgs Boson. These methods have been used to distinguish between signal and background events in high-energy physics, demonstrating how AI can aid in the discovery of fundamental particles (CERN, 2015 ).
Key Takeaways for Business Leaders
The work of Hopfield and Hinton highlights the potential of machine learning and AI to transform industries. Their journey underscores the importance of interdisciplinary thinking, showing how AI can enhance decision-making, improve efficiency, and drive innovation across sectors.

Business leaders should consider the ethical implications of AI, such as data privacy and algorithmic bias, and align AI tools with broader company goals. Leveraging AI effectively requires a strategic vision for using these technologies responsibly and innovatively.
The achievements of Hopfield and Hinton remind us that innovation often comes from exploring the intersections of different fields.
For businesses, fostering a culture of cross-disciplinary collaboration and supporting R&D can lead to breakthroughs that drive growth and keep companies competitive. Embracing interdisciplinary innovation helps companies solve current challenges and prepare for future disruptions.
Their determination to challenge paradigms and pursue ambitious research goals serves as a powerful reminder of the value of persistence in driving progress. Companies that cultivate a similar culture of exploration are more likely to achieve breakthroughs and create lasting value for their stakeholders.
Grand Thera is a technology company specializing in Data Science and AI, with over 10 years of expertise in technology and 20 years in venture capital. Our mission is to enable efficient data use across organizations of all sizes by aligning strategy and business with modular, adaptive solutions. This approach allows clients to achieve sustainable, competitive growth in today’s complex markets.
Comments